Understanding Image Annotation in the Business Landscape
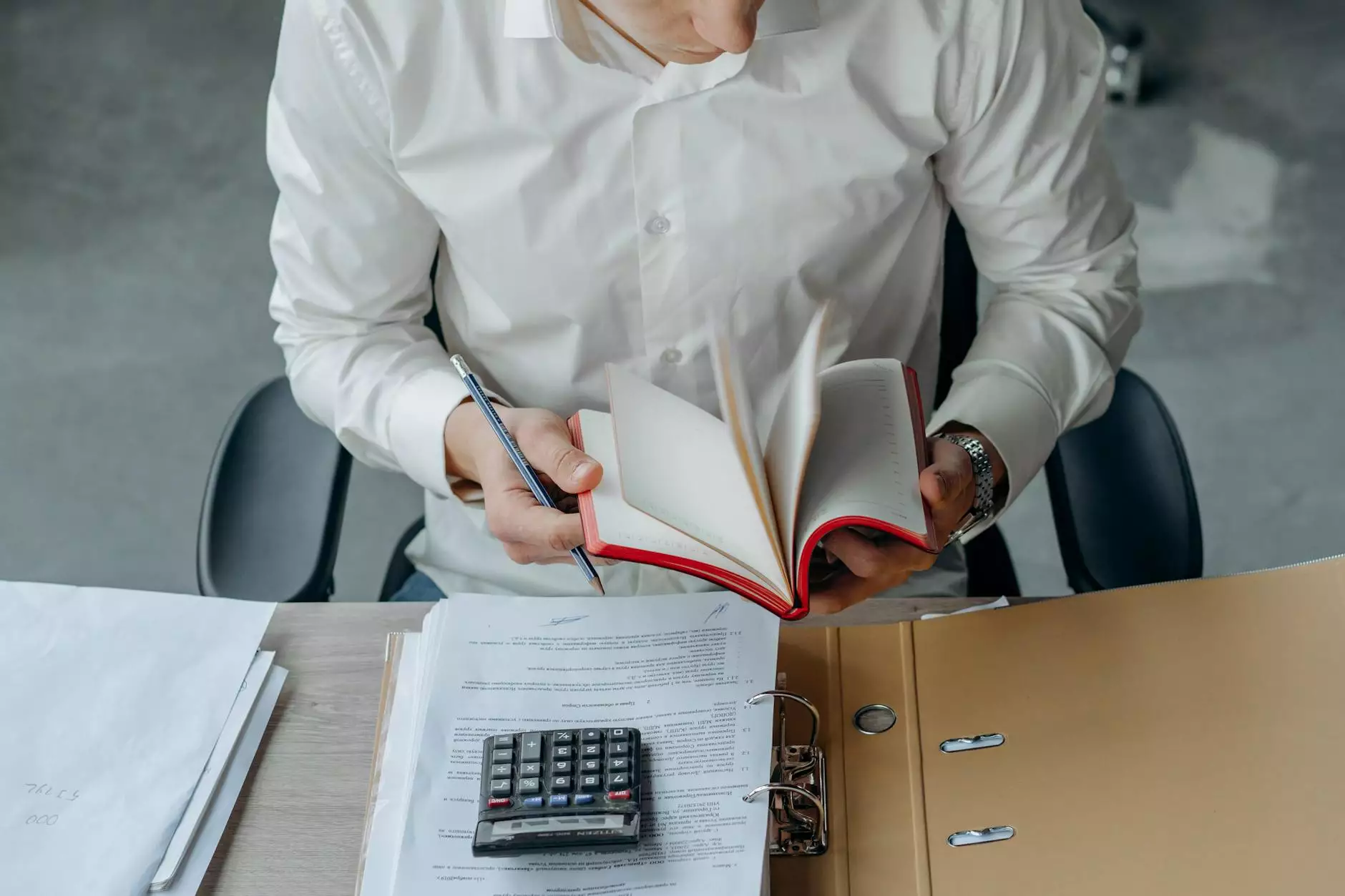
In the rapidly evolving digital world, businesses are constantly seeking innovative solutions to streamline operations, improve data handling, and ultimately, enhance their bottom line. One such innovation that is becoming increasingly essential is image annotation—the process of labeling and tagging images for a variety of applications ranging from machine learning to autonomous driving. In this article, we will delve deep into what image annotation is, the different types and techniques associated with it, and how leveraging a robust data annotation tool can propel your business forward.
What is Image Annotation?
Image annotation refers to the process of adding descriptive labels to images in order to train machine learning algorithms in image recognition tasks. This technique allows machines to understand and interpret visual information in a manner similar to humans. The annotations can come in various forms such as bounding boxes, polygons, and semantic segmentation, which help define and classify objects within an image. This practice is crucial in industries such as autonomous vehicles, healthcare imaging, and e-commerce, where data-driven decisions are paramount.
The Importance of Image Annotation in Business
As businesses strive for greater efficiency and accuracy in their operations, the role of image annotation becomes apparent. Here are several reasons why investing in high-quality image annotation is valuable:
- Enhanced Data Accuracy: High-quality annotated images enable businesses to train their algorithms effectively, resulting in superior accuracy in data-driven decisions.
- Improved Machine Learning Models: Accurate annotations are fundamental to developing and refining machine learning models that depend on image recognition, ultimately advancing AI technology.
- Scalability: Automated image annotation tools can process large volumes of data swiftly, allowing businesses to scale their operations without compromising quality.
- Competitive Edge: Companies that effectively utilize image annotation can gain a competitive advantage in their respective fields, harnessing the power of AI to enhance their offerings.
Types of Image Annotation
There are several methods of image annotation, each serving different purposes depending on the context of use. Here are the most common types:
1. Bounding Box Annotation
This method involves placing a rectangular box around the object of interest in an image. It’s one of the simplest and most common forms of annotation used for tasks such as object detection.
2. Polygon Annotation
In instances where the object shape is irregular, polygon annotation is preferred. Here, annotators draw a polygon around the object, providing a more precise capture of intricate shapes.
3. Semantic Segmentation
This process involves classifying every pixel in an image, enabling a model to understand the context of the entire image rather than just the objects. This technique is critical for tasks such as medical imaging where fine detail is essential.
4. Landmark Annotation
Primarily used in facial recognition, landmark annotation involves marking specific points on an image, such as the corners of eyes or the tip of the nose, to help models learn facial features.
5. Keypoint Annotation
This method focuses on marking specific key points on an object, allowing for detailed analysis of movements and actions, particularly relevant in the fields of sports analytics and human motion tracking.
The Role of Data Annotation Tools
To implement effective image annotation processes, businesses need to leverage specialized data annotation tools. These tools facilitate the annotation of images efficiently and accurately. Below, we explore some key features and benefits of using a dedicated data annotation tool:
1. User-friendly Interface
Most annotation tools provide an intuitive interface that simplifies the annotation process for users, regardless of their technical background. This ease of use leads to less time training personnel and more immediate productivity.
2. Automation Capabilities
Advanced annotation tools utilize machine learning to automate parts of the annotation process, significantly reducing the time and human effort required. This ensures that large datasets can be annotated quickly and with fewer errors.
3. Collaboration Features
Many modern annotation platforms support real-time collaboration, allowing teams to work on projects simultaneously. This feature is crucial for businesses with remote teams or those working on complex projects requiring multiple contributors.
4. Quality Control Mechanisms
Quality control is paramount in data annotation. Robust tools typically include features such as manual review processes and consistency checks to uphold the quality and reliability of annotated data.
5. Integration with Machine Learning Frameworks
A well-designed annotation tool can seamlessly integrate with popular machine learning frameworks and environments, ensuring that the transition from data collection to model training is smooth and efficient.
Choosing the Right Data Annotation Platform
With numerous options available in the market, selecting the right data annotation platform can be challenging. Here are some key factors to consider:
- Specific Needs: Assess your business's annotation needs—whether you require bounding box annotations, polygonal shapes, or semantic segmentation—and choose a platform that specializes in those areas.
- Scalability: As your business grows, your data annotation needs will evolve. Opt for a platform that can easily scale up and handle increased workloads.
- Cost-effectiveness: Evaluate pricing models and choose a solution that offers the best value without compromising on features and quality.
- Customer Support: Reliable customer support can make a significant difference, especially when integrating a new platform into existing processes.
The Future of Image Annotation and Business Applications
The future of image annotation is bright, with advancements in artificial intelligence, computer vision, and machine learning driving growth in this domain. As businesses increasingly rely on data to make informed decisions, the demand for accurately annotated images will only rise.
Applications of image annotation are vast and varied, including:
- Autonomous Vehicles: Annotated images are essential for training self-driving cars to recognize and react to their surroundings effectively.
- Healthcare: In medical imaging, precise annotations can aid in diagnostics and treatment planning, enhancing patient care and outcomes.
- E-commerce: Retailers can use image annotation to improve product recommendations and enhance user experience on their platforms.
Conclusion: Maximizing Business Potential with Image Annotation
In conclusion, the significance of image annotation in modern business cannot be overstated. As we move towards a more data-driven economy, harnessing the power of accurately annotated images will set businesses apart from the competition. By choosing the right data annotation tools and platforms, organizations can transform raw data into actionable insights, driving growth and innovation in their respective fields.
At Keylabs.ai, we understand the importance of image annotation and offer cutting-edge data annotation tools and platforms designed to meet the needs of businesses in various sectors. Join us as we embark on this journey towards enhanced efficiency and automation.